How Machine Learning Can Improve Security Gaps in Microsegmentation?
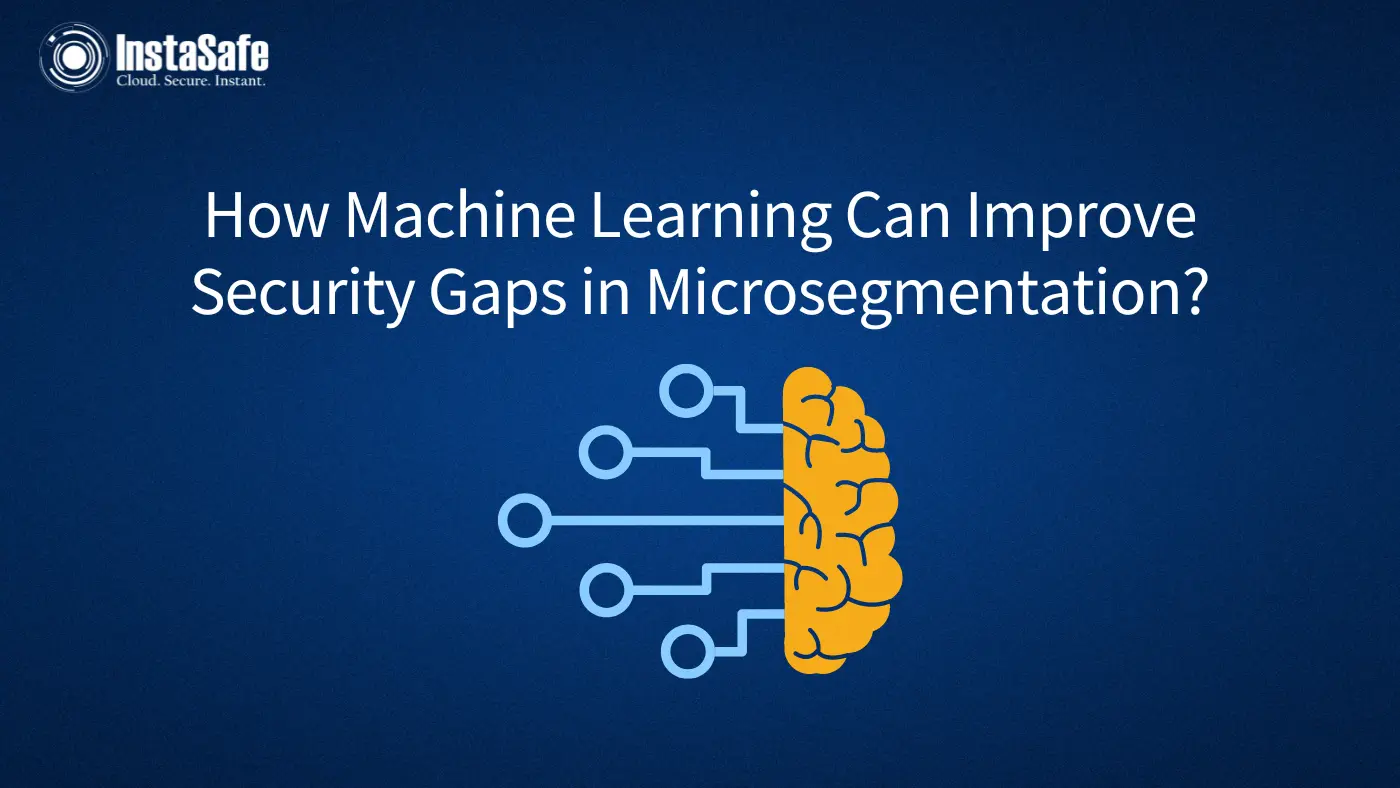
In its attempts to make enterprise security more granular and better, the DevSecOps sector is always teeming with industry disruptions. And, micro-segmentation is one such disruption.
Apart from making networks as granular as possible, the technique divides the entire enterprise network into small isolated segments that can be monitored and controlled more efficiently.
As the entire network is split into numerous segments with fine-grained control, management, and monitoring, the impact of a cybersecurity attack gets dispersed. While the premise of reducing the attack surface by network segmentation gives a promising way to ensure more safety and security of data and resources, it doesn’t come with security and performance gaps.
Here, we discuss how Machine Learning can address the security gaps in microsegmentation and how enterprises can leverage the technology at scale.
Let us begin with a brief overview of micro-segmentation.
Exploring Microsegmentation – Types and Operational Issues In a Non-Automated Environment
1. Network-Based Micro-Segmentation
The implementation of micro-segmentation is done on the network layer, and VLANs are used to create isolated segments. The user policies are configured and enforced on the basis of IP constructs or ACLs. Smaller networks can also leverage segmentation firewalls, but network-based micro-segmentation can create network bottlenecks and increases complexity.
So, it cannot be used for fine-grained micro-segmentation.
2. Hypervisor-Based Micro-Segmentation
In this approach, the hypervisor is used to create isolated segment workloads. It is easier to implement as all the network traffic already passes through the hypervisor. The security professionals can enforce policies outside the workload in an agile manner.
However, the process visibility is affected and the number of policies supported by the hypervisor is lesser than the other techniques. Further, this approach is liable to vendor lock-ins.
3. Host-Based Micro-Segmentation
Here, micro-segmentation is implemented via a software-defined framework. The technique employs the native firewall functionality of the built-in workloads for highly distributed granular policy controls.
However, this approach also has some cons – it cannot be implemented without an agent on each host and IT teams require more awareness for this, as it is a newer technique, as compared to traditional infrastructure techniques.
Challenges of Manual Micro-Segmentation: Why Do We Need ML in the Picture?
By now, you might have realized that manual or non-automated micro-segmentation is more of an overhead and complex process than it seems.
- Manual micro-segmentation consumes a lot of time, effort, and resources because East-West communications are more in number as compared to North-South communications. Hence, more policies must be created and managed.
- Further, you need to establish proper ownership in your organization and decide the roles and responsibilities for segmenting the network.
Whether it falls under the security department or the network department?
Figure out the answer as soon as you can, and it is – Enterprise security is always a shared responsibility!
- Also, never disregard the state of your private physical data centre, and opt for a virtual data centre for micro-segmentation. This can spur policy discrepancies over time and lead to misconfigurations, ultimately birthing breaches!
Hence, Automation!
Good Read: How Artificial Intelligence (AI) Plays an Important Role in Cybersecurity
Automated Micro-Segmentation: How Can Machine Learning Address the Gaps?
In enterprise networks, proper implementation of micro-segmentation requires:
- Discovery of all workloads
- Creation of application-dependency mappings
- Workload and Label classification
Machine Learning can not only make the entire process faster and more efficient but also deliver many smart functionalities and offer actionable insights into the overall health of all the segments.
AI-based automation emerges as a commendable and reliable agent to ensure that you get the most of your micro-segmented network, without having to embroil in the technicalities and thread/workload policy management.
Automatic Discovery
ML will collect all the information about every flow in the enterprise network. Automatic discovery and visualization of all the app dependencies and app communications make the entire process of workload management easier and more efficient.
The data from this visualization and information flow discovery is then fed to the different algorithms to create a network graph and leverage the ML for creating clusters of similar workloads.
So, with ML-based micro-segmentation, you can have:
- Automated workload classification
- Automated rule suggestions
- Automated label creation for apps
- Policy Simulation
ML facilitates real-time and historical policy simulation and also offers smart auditing and policy validation. This will allow you to detect and view the policy changes over time in the form of a graph, and you can take proper actions.
This comes in handy in many other situations such as impact assessment of policy validation in real-time. You can see which segments are negatively impacted and what aspects are impacted.
Such granular insights with actionable data stemming from minute changes are impossible in manual micro-segmentation.
Continued Learning and Adaptation
ML-based micro-segmentation continues to learn and adapt to changes, risks, vulnerabilities, and experiences it earns over time.
It can optimize policies with respect to changes in the security structure of your enterprise and enforce an intended state of security over the segment components (workloads).
The manual micro-segmentation comes with a bit of anticipation and estimation of the security professionals, and it is a Herculean task to continuously learn and adapt as per the attacks or changes in security.
Further, once micro-segmentation is done, manual management, error-free monitoring, and enforcement are not as stringent and efficient as the ML.
Hence, ML can be leveraged on three distinct levels for making micro-segmentation a delightful success:
- Data – It can monitor the entire enterprise network at the app level.
- Objective – It can monitor the network traffic and automatically discover the information flow in it.
- Algorithm – It can create user-friendly rules that are neither overwhelming nor compromising with the security constructs of the network.
How to Apply Machine Learning for Micro-Segmentation in an Enterprise Network?
Identify and Define the Intended Security State of the Enterprise Network
Find out what type of security state you wish for the entire network and specific network segments. Discuss the various access rights and permissions that you must enforce, how thorough and detailed the validation checks must be, and what your micro-segmented network should look like?
Once you are ready with the basic instructions and a rough idea of how your network segments or workloads should work, you are ready for the next step.
Define the Security Constructs and Optimized Security Policies to Be Enforced
Once you know what you want your network to look like, you have to define the optimized policies for maintaining the network in that state.
In simpler words, you define the rules that your entire network and all the micro-segments have to follow, to ensure that system security is not violated or gets deviated from the ideal state.
This enforcement becomes the duty of the ML part of your network, as outlined earlier.
So, you can think of this part as filling “specifications” in a builder form.
Learning, Adapting, and Optimizing the Security Policies
Once you have created a micro-segmented network, you have to ensure that its security state remains as is. However, the attacks are becoming more and more sophisticated and well-resourced attackers can also leverage ML via adversarial examples or fake behavioral inputs.
So, the ML needs to consistently learn from the network, external actors, and attacks. Based on these learnings, it needs to adapt the micro-segments and optimize its security policies to ensure that the safety and security of the enterprise network do not falter.
Machine Learning for Micro-Segmentation: Results Are Driven by Right Tools
Okay, so now we now know that ML-based micro-segmentation is a reliable and robust way to keep your enterprise network safe from attacks and to minimize their impacts.
However, Machine Learning is still evolving and is continuously drizzled with disruptions. So, choosing the right provider or vendor is of critical importance!
InstaSafe, a leading Zero Trust security solutions provider, offers a highly intuitive, smart, and efficient way to leverage ML for micro-segmentation.
It offers segmentation in various ways:
- Vulnerability-based Segmentation
- Application-based Segmentation
- User-based Segmentation
- Location-based Segmentation
For more information and for effortless ML-based micro-segmentation, schedule a product demo NOW!
Popular Searches
Biometrics Authentication | Certificate Based Authentication | Device Binding | Device Posture Check | Always on VPN | FIDO Authentication | FIDO2 | Ldap and SSO | Multi Factor Authentication | Passwordless Authentication | Radius Authentication | SAML Authentication | SAML and SSO | What is Sdp | Devops Security | Secure Remote Access | Alternative of VPN | Zero Trust VPN | Zero Trust Security | Zero Trust Network Access | ZTAA